Real World Evidence
Robust Causal Inference
Inferring about Treatment Effect with EHR via Drugable Genome
Linking EHR with RCT
Individualized Treatment Effects with Observationa Data
Research Team
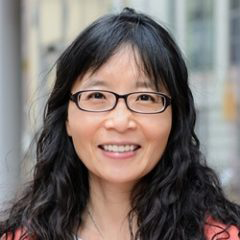
Cai, Tianxi
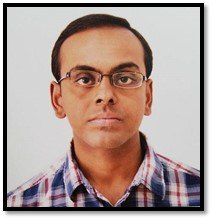
Mukherjee, Rajarshi
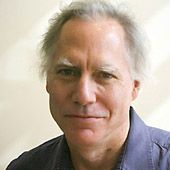
Robins, James
Selected Publications
L Liu, R Mukherjee, JM Robins, 2019, “On assumption-free tests and confidence intervals for causal effects estimated by machine learning”, https://arxiv.org/abs/1904.04276.
Greenland, S., Fay, M.P., Brittain, E.H., Shih, J.H., Follmann, D.A., Gabriel, E.E. and Robins, J.M., 2019. On Causal Inferences for Personalized Medicine: How Hidden Causal Assumptions Led to Erroneous Causal Claims About the D-Value. The American Statistician, pp.1-11.
D. Cheng, A. Ananthakrishnan, and T. Cai, 2019 “Estimating Average Treatment Effects with a Double-Index Propensity Score.” Biometrics, in revision.
D. Cheng, A. Ananthakrishnan, and T. Cai, 2019 “Efficient and Robust Semi-Supervised Estimation of Average Treatment Effects in Electronic Medical Records Data.” Biometrics, in revision.
T. Cai, T.T. Cai, Z. Guo, 2019 “Individualized Treatment Selection: An Optimal Hypothesis Testing Approach In High-dimensional Models”. https://arxiv.org/abs/1904.12891.
T. Cai, Y. Zhang, Y. Ho, N. Link, J. Sun, J. Huang, T.A. Cai, S. Damrauer, Y. Ahuja, et al., 2018. “Association of interleukin 6 receptor variant with cardiovascular disease effects of interleukin 6 receptor blocking therapy: a phenome-wide association study.” JAMA cardiology, 3(9), pp.849-857.
Y. Xia, T. Cai, T.T. Cai, 2018 “Two-Sample Tests for High-Dimensional Linear Regression with an Application to Detecting Interactions”. Statistica Sinica, 28(1), 63-92.